Deep learning has been a hot topic over the last couple of years, especially given the fear of the destructive power of artificial intelligence and machine learning. However, few people these days know that the term itself was introduced back in 1986. Rina Dechter used it in her article Learning While Searching in Constraint-Satisfaction Problems. In the paper, deep learning was interpreted as the act of “acquiring all the possible information out of a dead-end.” Time passed, and the concept grew far more complex than that. However, the idea of gathering all possible knowledge from the source remained fundamental to how the technology works. So, what is deep learning in the modern sense?
Deep learning definition
Deep learning is the concept defined today as computational intelligence used for gathering knowledge, learning from this experience, and building out complicated concepts from simple ones. If you wonder whether deep learning is a subset of machine learning, the answer is yes. As a field of ML and a subfield of AI, deep learning focuses on modeling the human brain in the context of data collection and data analysis.
Deep learning is referred to this way because neural networks that it uses to learn have various deep layers. Brought in in the 1950s, these networks were an attempt to simulate the network of neurons that allow a human brain to interpret the context of real-world situations and make decisions based on that. Today, they are the central unit of every machine learning system, from search algorithms to pattern recognition and object detection. But this neural network architecture isn’t as easy to understand as it may seem.
How deep learning technology works
The basic idea of artificial neural networks is to train the system by feeding it lots of data called a training set. As the network "learns" the basic rules, the training process is adjusted to become more efficient. A regular Deep Neural Network (DNN) is basically a set of algorithms designed to recognize patterns, cluster and classify data.
Besides, deep learning systems have their own environments where the task is to create a neural network to analyze different types of data like images, sounds, or texts. Within these environments, neural networks use different layers of mathematical processing to make sense of information received from the outside world. Stacked layers of neurons, which form a network, allow input units to pass through them in order to transform incoming data and provide the correct output.
For instance, if we need the network to recognize speech, we expose it to a vast amount of data about speech patterns and vocalizations of many speakers. And once we have trained the network to recognize particular speech patterns, it is then able to identify and understand the speech of any person itself. In that sense, deep learning technology is learning what other people sound like.
Now, let’s just look at one example to get a quick sense of how this works. Imagine that we have a deep learning system that learns that the difference between "wow" and "awww" is minor. If we feed this system 100 people’s audio files and ask it to classify one of them as either "wow" or "awww," it will very quickly detect which is which. Moreover, it will be capable of detecting this difference in a person’s voice at much higher accuracy than humans can do. So, these were the definition of deep learning and its basic principles.
What is deep learning technology business potential?
Suffice it to say, deep learning has the potential to lead to the development of more efficient, business-oriented software and systems, offering a large amount of value to people. And the best way to understand a deep learning system is through drawing and analyzing the real-life examples of solutions that are using deep learning technology. Below are some of the tasks successfully supported by deep learning technologies.
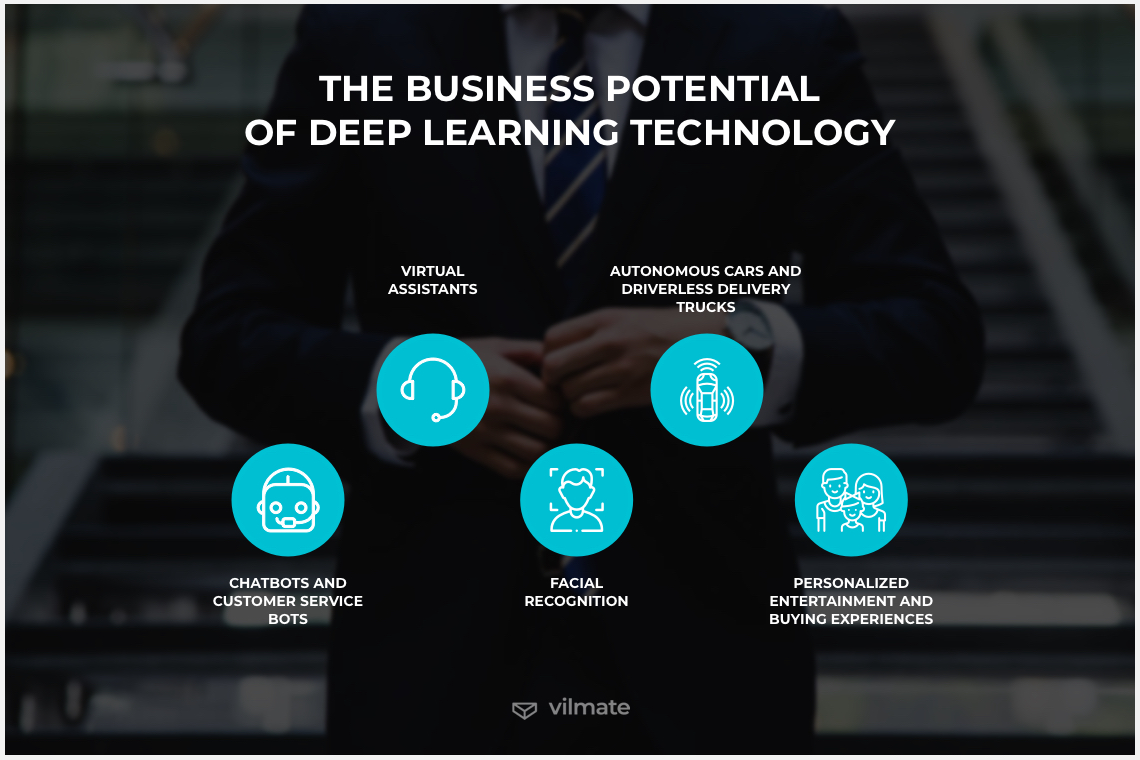
1. Chatbots and customer service bots
Chatbots that are using AI and deep learning, particularly, are widely agreed to be one of the biggest web development trends in 2020. With the rise of deep learning, chatbots are becoming progressively human. They have the potential to become even more engaging and capable of providing efficient customer support and more personalized responses.
A deep learning chatbot differs from a regular one in a level of human-likeness and sophistication. A deep learning chatbot learns from data and human-to-human dialogues. Meanwhile, regular chatbots, to a higher degree, rely on the input of human programmers to function. In the case of the AI-based chatbots, programmers do not determine how the received data is interpreted. The deep learning system can come to its own conclusions and give answers to some questions emulating human performance. This is why the technology has the potential to be put to good use in the field of customer service.
2. Virtual assistants
A deep learning-based virtual assistant like Alexa or Siri could be an ideal way to let users complete tasks with voice. Virtual assistants are great for routine tasks, while consumers can expect even more interactive capabilities when these are coupled with the internet of things. For example, Alexa would allow a homeowner to remotely unlock a door, stream music to an A/V receiver in a bedroom, or turn on the TV.
Deep learning is a key factor in making all this happen. Of course, the use of large datasets (e.g. of voice recordings) is essential to facilitate proper training with hundreds of thousands of examples. Deep learning technology is very good at finding regularities, especially considering that people tend to keep saying the same things. So, regardless of how complex you think the task is for a virtual assistant, it will be efficiently performed.
3. Facial recognition
Deep machine learning algorithms for facial recognition are commonly known to have security reasons behind them. Deep learning methods can leverage massive datasets of faces to perform or even outperform the face recognition capabilities of humans. Essentially, this technology helps verify or identify a person. There is a set number of steps in a face recognition processing flow that are fundamental to how Face ID works:
- Face detection
- Face alignment
- Feature extraction
- Feature matching
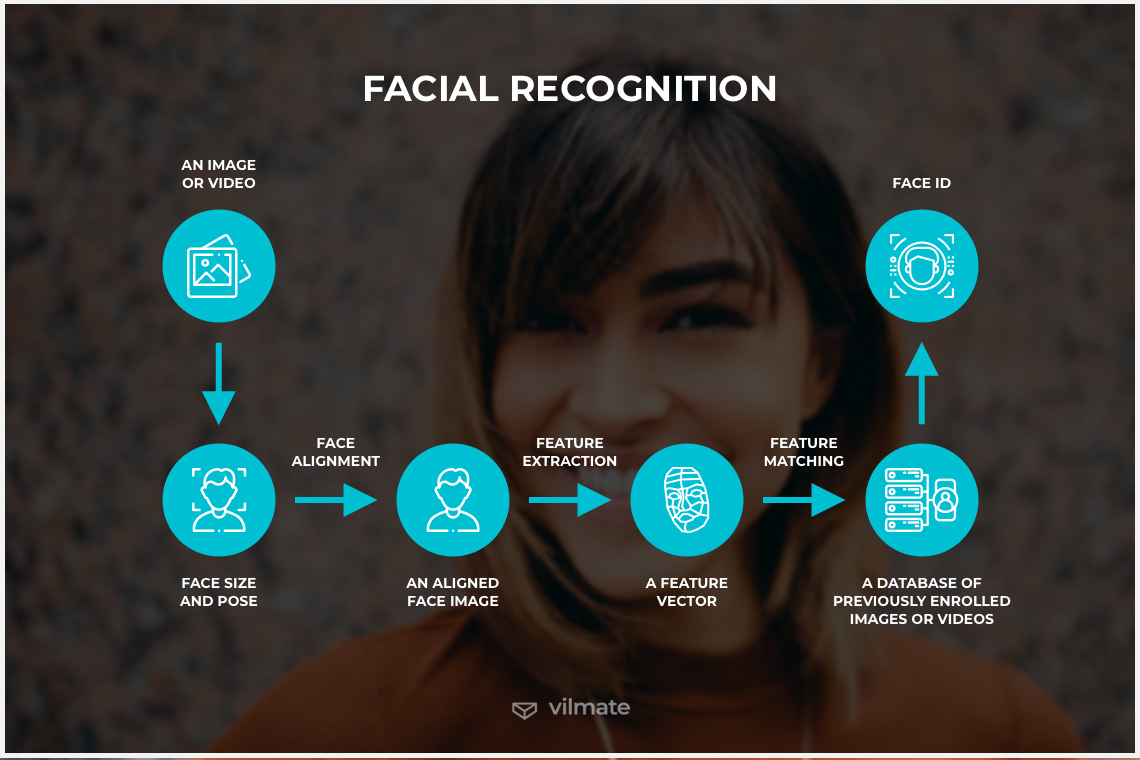
Similar to other deep learning systems, a face recognition system is trained on samples with inputs and outputs. Most often, these are photos with at least one face in them. Deep convolutional neural networks allow stacking these photos into a more structured dataset so that the system can become more accurate. For example, Facebook uses artificial neural networks for its DeepFace algorithm. This algorithm is claimed to be able to recognize specific faces with 97% accuracy.
4. Autonomous cars and driverless delivery trucks
Self-driving cars are another deep learning achievement. The point is to give the machines as much context as possible about their environment. Self-driving vehicles could use machine learning to predict when to take action. They can understand the realities of the road and how to respond to them appropriately. Again, the data is king. Computer vision systems can be applied to all sorts of car sensors to visualize the world. But to become progressively more human-like in their information-processing and decision-making, the algorithms need more data to train on and thus be able to distinguish between pedestrians and similar-looking objects or differentiate between traffic lights and lights indicating a rush hour.
Not only such big names as Tesla and Alphabet use industry-leading AI and deep learning technologies. There are many other organizations investing in autonomous R&D. Ford, Honda, Huawei, Hyundai, and quite a number of other companies are concerned with this new frontier.
5. Personalized entertainment and buying experiences
Online retailers already use deep learning to improve recommendations for customers or enhance the search experience returning better results to queries. Deep learning algorithms can also support visual search. Thus, when a customer sees an item of clothing in the real world, for example, he or she can find it via visual search. The deep learning system will identify the article by an image and locate an online store for a customer to purchase this thing.
A higher level of personalization provided by deep learning tech can also be achieved in the entertainment arena. The technology enables learning algorithms to analyze the content a user consumes. Everybody knows how excellent streaming services like Netflix at recommending things that match perfectly the viewer’s interests.
Conclusion
Presently, deep learning technology is still in its infancy. However, it already has many amazing and interesting applications across a breadth of industries. It can do speech recognition, speech to text translation, image processing, colorization of black-and-white images, etc. So, here has never been a better time to become a part of it.
Now that you have a clearer idea of what deep learning is and how it works, it must be easier to understand the current and future capabilities of deep learning technology and see what to expect from the next revolution in computing.
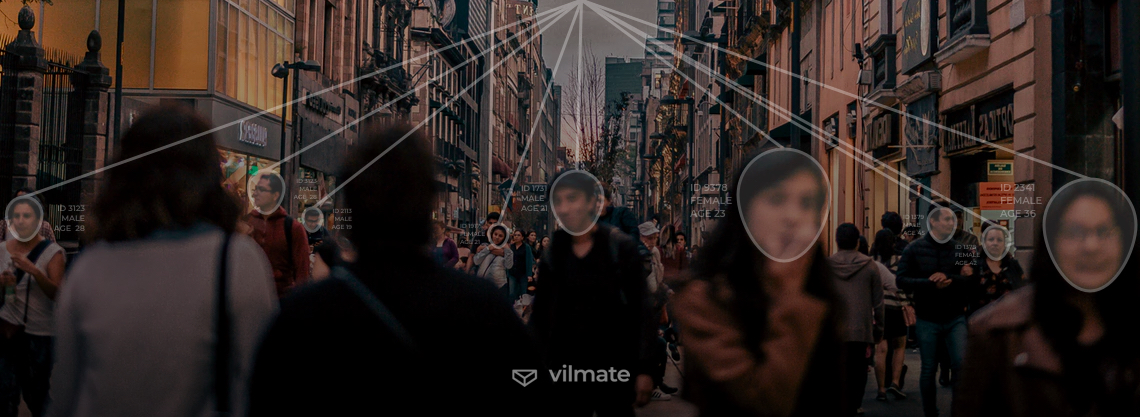
© 2020, Vilmate LLC